6G-SANDBOX
Next cell prediction using Graph Neural Networks (NEXT-CELL-GNN)
NEXT-CELL-GNN aims to deliver scalable and performant GNNs that can predict user connections in cellular networks while fulfilling network operators' accuracy and inference requirements. Several challenges arise for such an experimental analysis to address, such as: What kind of L1/L2/L3 metrics could be of value for training GNN models? How can raw L1/L2/L3 metrics be transformed in graph-structured data so they can be used to train different GNN models? What node features are of most importance for different GNN models? What modifications/extensions of existing GNN models (e.g., GCNs, graphSAGE, GATs, VGAE, SEAL) are needed to support accurate and fast predictions of user connections in cellular networks? How can telecom GNN models be optimised to improve their performance?
Overall, NEXT-CELL-GNN aims to push the boundaries of intelligent network operation in the Next-Cell prediction use case, paving the way for fast and efficient GNN models in the future AI-based 6G ecosystem.
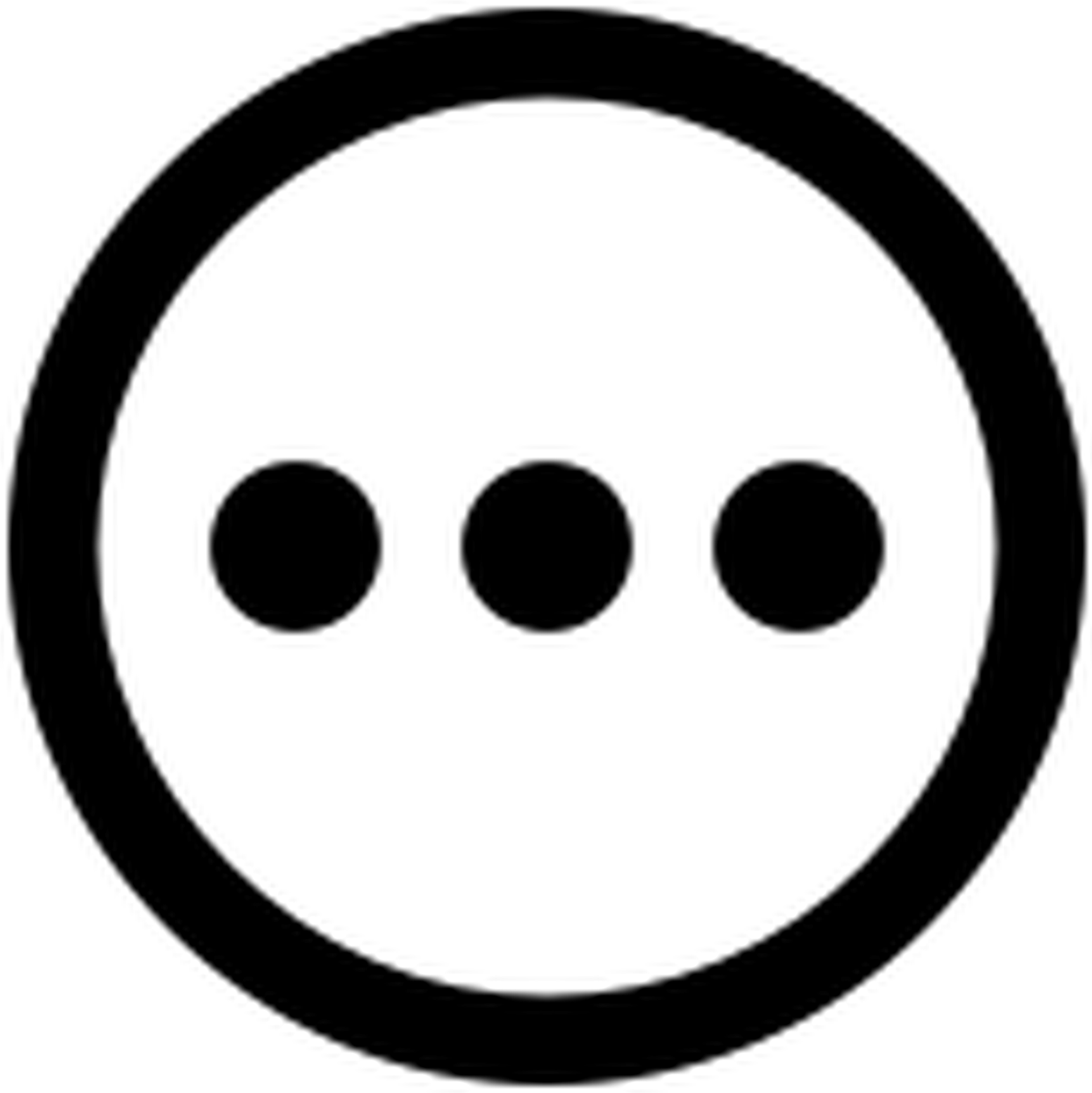
Project Open Call 3rd-party funding
6G-SANDBOX
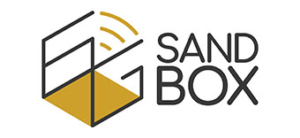